24 NOV, 2023 | by Nilesh Dherange
8 Nov, 2023 | By Nilesh Dherange
The 3 key stages of ransomware attacks and useful indicators of compromise
24 Oct, 2023 | BY SARYU NAYYAR
18 Oct, 2023 | by Craig Cooper
From Trust to Zero Trust: The Role of Identity Analytics and ITDR
17 Oct, 2023 | by Byron Acohido
SHARED INTEL Q&A: Everything the Cisco-Splunk merger tells us about the rise of SIEMs
17 Oct, 2023 | by Saryu Nayyar
How To Develop A Plan For Bringing Generative AI Into Your Business
5 Oct, 2023 | by Amol Bhagwat
How to Unify Multiple Analytics Systems to Determine Security Posture and Overall Risk
9 November, 2023 | forbes.com
Assisting The Unsung Heroes Of The SOC: Using Automation To Accelerate Threat Investigations
31 October, 2023 | forbes.com
20 Tech Pros Share Red Flags To Watch For Before Sharing Personal Data
16 October, 2023 | e-channelnews.com
12 October, 2023 | forbes.com
Small-Business Cybersecurity: 20 Effective Tips From Tech Experts
28 SEPTEMBER, 2023 | forbes.com
20 Women Tech Leaders On The Principles That Guide Their Leadership
27 September, 2023 | crn.com
14 September 2023 | channelfutures.com
FEATURED BLOG POST:
Gurucul is a Top 3 SIEM
CURRENT REPORT:
2023 Insider Threat Report
LATEST WHITEPAPER:
Improving Data Ingestion While Decreasing Complexity and Cost
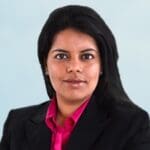
Read all Forbes Technology Council articles from our CEO, Saryu Nayyar